For AI companies, bias in data collection is a big issue.
Why?
Here’s the bottom line: “Bias in data produces biased models which can be discriminatory and harmful to humans”. – source
Amazon, for example, shut down a data model that penalized women in only 2018, and Google’s ad ranking systems have been accused of gender profiling for over 5 years.
The reason for the problems? The sources of data are used to train them.
To combat bias for good, we’re going to take you through the different types of biases you should be aware of in data collection. After identifying and correcting data bias, you will have a data model that is sure to accurately, and ethically, reflect your investigation.
What types of Bias are in AI?
Bias in AI can be categorised into two main types: cognitive biases and lack of complete data.
Cognitive Biases
Cognitive biases are unconscious errors in thinking that affect individuals’ judgments and decisions. These biases can seep into machine learning algorithms through two primary sources:
- Designers’ Unconscious Biases: Designers may unknowingly introduce their own biases into the model during the algorithm development process. These biases reflect their personal beliefs, opinions, and experiences, which can influence the model’s behaviour.
- Biased Training Data: The training data used to train AI algorithms may contain biases. These biases stem from societal prejudices and can manifest in the form of gender bias, racial bias, or other forms of discrimination. When the training data includes these biases, the AI model may unintentionally perpetuate discriminatory outcomes.
Lack of Complete Data
Another source of bias in AI is the lack of complete data. If the data used for training the AI system is not representative of the entire population, it may introduce bias. For example, research studies in psychology often rely on results from undergraduate students, which may not adequately represent the diversity of the general population.
What types of bias are there?
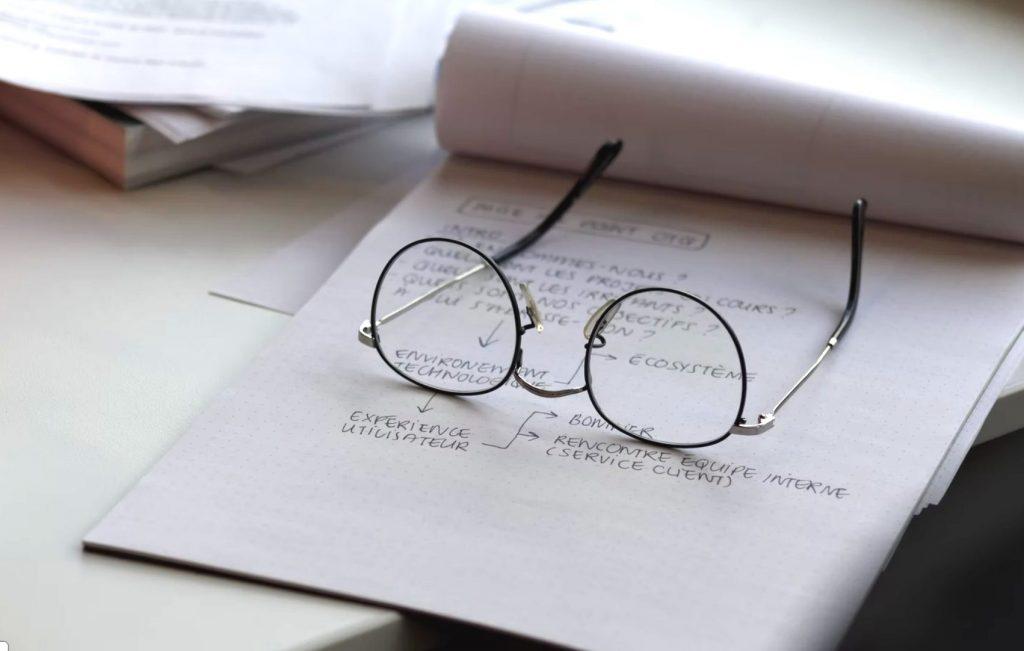
Response/Activity Bias
This specific type of bias occurs in user-generated data, i.e. posts on social media (Facebook, Twitter, Instagram), reviews on eCommerce websites, etc.
As the people who contribute to user-generated data are a small percentage of the entire population, it is likely their opinions/preferences will reflect the opinions of the majority.
Societal Bias
Societal bias is generated purely by content produced by humans. Whether this is on social media or curated news articles, this type of bias still exists. An instance of this is in the use of gender, or race stereotypes. This can also be known as label bias.
Omitted Variable Bias
This is the bias that occurs in data when the critical attributes, that influence its outcome, are missing. Usually, this happens when data generation relies on human input, allowing more room for mistakes. This can also happen through the recording data process not having access to key attributes.
Feedback Loop/Selection Bias
This bias involves the model influencing the data that is used to train it.
Where does this occur? Often, selection bias takes place in rank content (think ad personalization, etc), by presenting items to certain users, over others.
The labels for these items are then generated by the users’ responses to the items that are collected. Items not collected are therefore left with unknown responses. User responses, however, can be influenced. They can be influenced by any element to do with the item – whether it’s its’ position on the page, the font, or the media.
According to Latanya Sweeney’s research on racial disparities in online ad targeting, searches for names associated with African Americans typically yielded more ads featuring the word “arrest” than searches for names associated with White-identifying names. Sweeney hypothised that even though the different ad copy versions with and without the word “arrest” were initially presented equally, consumers might have clicked on different versions more frequently for different searches, causing the algorithm to display them more often.
System Drift Bias
This type of bias occurs when the system that generates the data experiences changes over time. These types of changes include attributes captured in the data (including outcome), or changing the underlying model/algorithm so the user interacts with the system differently altogether.
Introducing new modes of user interaction (i.e., share buttons), or adding a search feature into your system, can be some of the many ways system drift bias can occur.
How to Identify Bias?
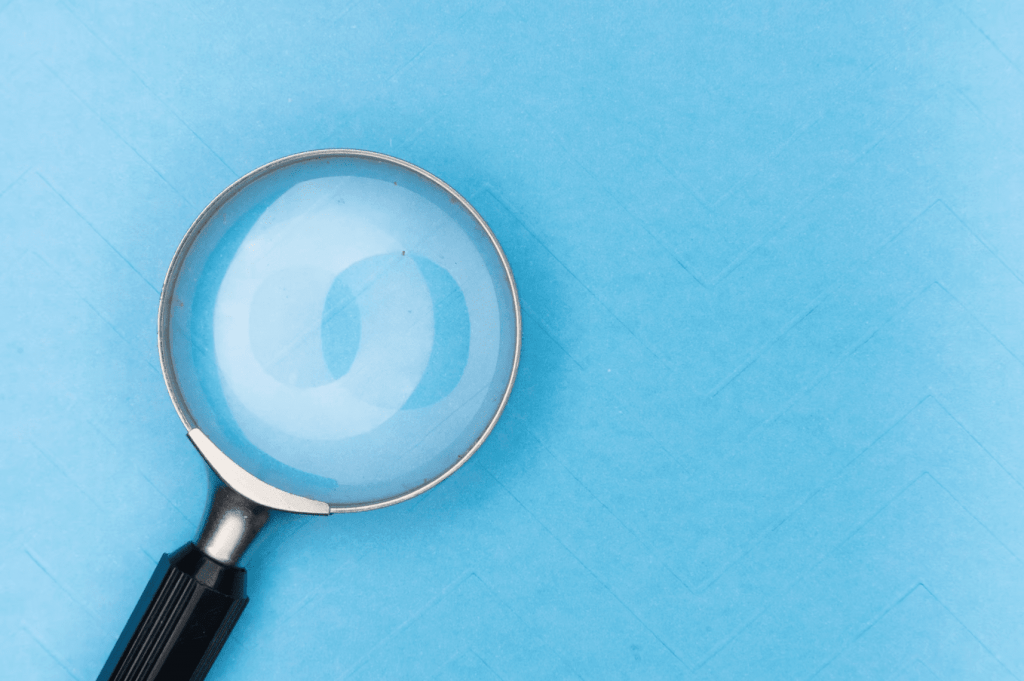
There are 3 instances where bias can introduce itself in an investigation:
Data collection
Data collection is one of the most common places to find biases. Why? Data is typically collected by humans, therefore lending more opportunity for error and bias. The common biases found in data collection can be categorized into:
- Selection Bias – the selection of data isn’t representative of the population as a whole and therefore presents a bias.
- Systematic Bias – a consistent error that repeats itself throughout the model.
- Response Bias – participants of data respond to questions in a way that is deemed false, or inaccurate.
Data preprocessing
This is where you prepare the data to be analyzed – think of this as an extra step in ensuring 100% ethical and unbiased data.
First, you will need to determine whether there are any outliers within the data, that would have an unnatural impact on the model itself.
Handling missing variables can also be a key indicator in introducing bias. If missing values are ignored, or instead replaced with the ‘average’ of data, you are effectively altering the results. Your data collection would then be more biased to results than reflecting the general ‘average’.
And sometimes, data is simply filtered too much! Over-filtering data can often have the effect of no longer representing the original data target.
Data analysis
Despite going through the two initial stages of data collection, you may still find bias within data analysis. These are the most typical biases seen in the analysis stage:
- Confirmation bias – involving preconceptions, and focusing on information that supports this theory.
- Misleading charts (or graphs) – a distorted display of information that incorrectly represents data. From this, an incorrect conclusion is formed based on the model.
Fixing Bias in AI and Machine Learning Algorithms
First and foremost, if your data collection is complete, you should recognise that AI biases are solely the result of human prejudice and concentrate on eliminating those prejudices from the data set. It’s not as simple as it seems, though.
Removing data that contains protected classes (like race or sex) and the labels that bias the algorithm is a basic method. However, this strategy could not be effective because the model’s comprehension could be impacted by the omitted labels, which would worsen the accuracy of your results.
While there is no quick fix for eliminating all biases, there are a few suggestions from consultants like McKinsey outlining the best practices to reduce AI bias:
1. Assessing Bias Risks in Algorithms and Data
To identify potential sources of bias, it is essential to thoroughly understand the algorithm and the data used for training. This involves examining the training dataset for representativeness, analyzing subpopulations, and will need to stay up to date with the model’s performance to determine how and where AI can improve fairness.
2. Implementing a Debiasing Strategy
Developing a comprehensive debiasing strategy is crucial to minimising bias in AI systems. This strategy should encompass technical, operational, and organizational actions. Technical strategies involve using tools to identify and mitigate bias, operational strategies focus on improving data collection processes, and organizational strategies involve creating a transparent and inclusive workplace culture.
3. Improving Data Collection Processes
Data collection is a common source of bias, as it involves human involvement and the potential for errors and biases. To minimise bias, it is essential to collect diverse and representative data. This can be achieved by involving diverse perspectives in the data collection process, conducting thorough data preprocessing, and avoiding over-filtering of data.
4. Enhancing Model Building and Evaluation
Throughout the model-building and evaluation process, it is crucial to identify and address biases that may have gone unnoticed. This involves continuously assessing the model’s performance, identifying biases, and making necessary adjustments. By improving the model-building process, companies can reduce bias and improve the overall accuracy and fairness of their AI systems.
5. Involving Multidisciplinary Approach and Diversity
Minimising bias in AI requires a multidisciplinary approach that involves experts from various fields, including ethicists, social scientists, and domain experts. These experts can bring diverse perspectives and insights to the AI development process, facilitating the identification and mitigation of biases. Additionally, maintaining a diverse AI team can help in recognising and addressing biases that may otherwise go unnoticed.
6. Leveraging Bias Detection and Mitigation Tools
Several tools and libraries are available to assist in detecting and mitigating biases in AI systems. For example, the AI Fairness 360 library developed by IBM provides metrics and algorithms to test and mitigate biases in AI models. IBM Watson OpenScale offers real-time bias checking and mitigation capabilities. Google’s What-If Tool enables the analysis of model behaviour and the importance of different data features. Leveraging these tools can aid in identifying and addressing biases effectively.
Final thoughts
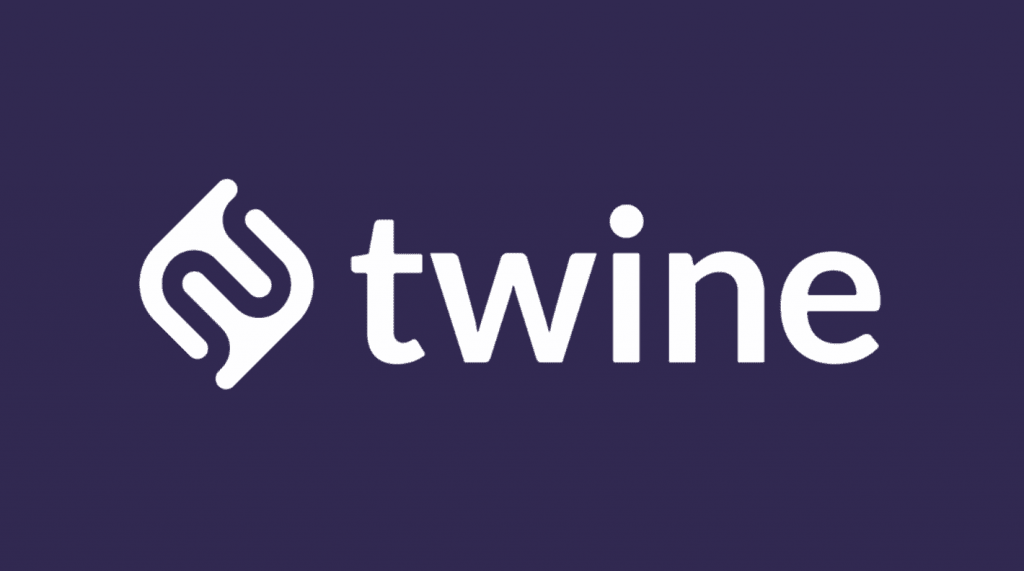
Once you have figured out the source of bias, you have successfully handled half of the battle. It is your decision on whether you remove the bias, or handle the bias.
For example, if there is a class imbalance within your model that makes it more biased, then you could look into ways of resampling.
Working with a reputable and ethical source, from the beginning, will eliminate any risks of bias within your AI data collection.
Twine can help build you a dataset that is free of bias.
From our global marketplace of over half a million diverse freelancers, we are able to provide a wide selection of data to build your model from the ground up. Our team will work on audio and video datasets, entirely customized to your requirements.