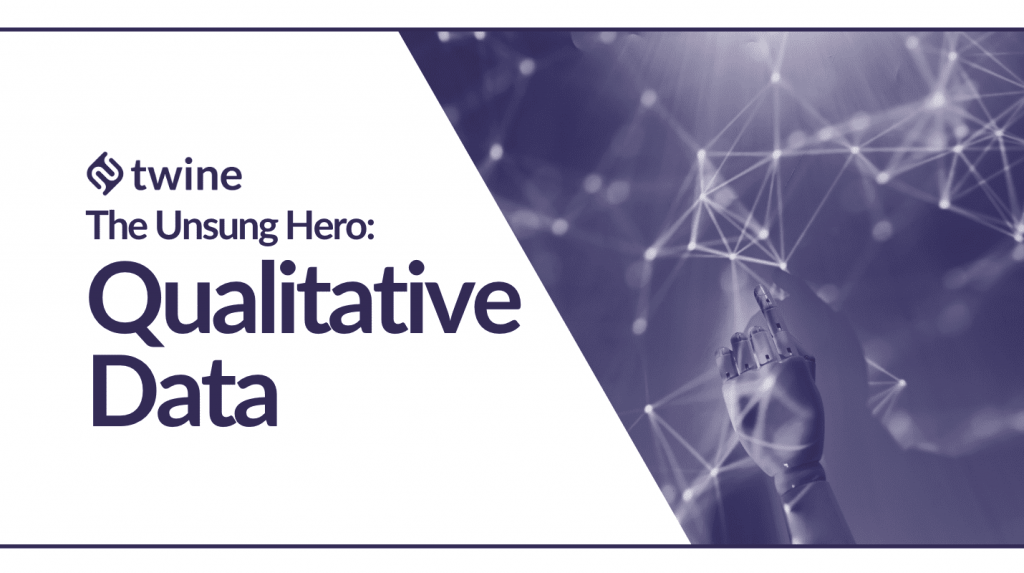
If you’re like most people, you probably think of machine learning (ML) as a black box that takes data on one end and spits out predictions on the other. But what if I told you that there’s a hidden hero in machine learning datasets – qualitative data?
If there’s one thing that all data scientists can agree on is that more data is always better but when it comes to machine learning datasets, not all data is created equal. If quantitative data is the bread and butter of ML datasets, then qualitative data might be thought of as delicious bacon.
In fact, the quality of your dataset can be the difference between a model that performs poorly and one that exceeds your expectations! While not as flashy as its quantitative counterpart, qualitative data is nonetheless a critical component of strong ML models, especially when it comes to speech and vision datasets.
In this article, we’ll discuss what qualitative data is, and why it’s important, and we’ll also highlight some key benefits of using ethically sourced qualitative data in your ML projects.
Without further ado, let’s get started!
Qualitative Data vs Quantitative Data
Qualitative data is a form of unstructured data that provides insights into the why and how of things. Unlike quantitative data, which relies on objectivity and measurement, qualitative data provides understanding through words or pictures.
It can be used to train machine learning algorithms by providing information about the structure and meaning of images or videos. This type of data is important for tasks such as facial and speech recognition, where context is critical for accurate results
Qualitative data can also be used to improve the performance of machine learning models by identifying errors and correcting them. With the increasing use of artificial intelligence in business, creative freelancing, ai copywriting, and even everyday life, it is becoming increasingly important to have access to quality datasets for training these systems.
Quantitative data, on the other hand, is structured data that relies on objectivity and measurement. It can be used to train machine learning algorithms by providing numerical values that can be analyzed statistically. Quantitative data is important for tasks such as predicting consumer behavior or stock prices, where precision is key. As an essential part of any machine learning dataset, quantitive data should not be overlooked!
Start growing with data and Twine AI
What are the Benefits of Qualitative Data?
Qualitative Data is a broad term that can be used to describe pretty much any type of data that isn’t numerical. In other words, it can include text, images, and audio recordings. This has a number of benefits that make it an important part of any ML dataset:
- Firstly, it can improve accuracy by providing additional context for the AI system.
- Secondly, it can make training datasets much smaller. This is particularly helpful for constrained devices like mobile phones.
- Finally, it can prevent AI systems from becoming “over-trained” on specific datasets, leading them to be less accurate when applied to new data.
One of the main benefits of qualitative data is that it is much easier to understand than quantitative data. This makes it perfect for feeding datasets into artificial intelligence systems like vision and speech recognition algorithms.
The human brain is very good at understanding natural language and visual information, so using qualitative data in these applications can result in better performance overall.
Another key benefit of this data type is that it is often cheaper to obtain than quantitative data. Qualitative data can be gathered from many resources online, making it more affordable for smaller businesses or start-ups that don’t have much financial backing.
Qualitative data also has a high rate of reusability. Because qualitative datasets are so easy to understand, they can be used multiple times in different applications with little modification required.
Reducing Bias
And finally, one huge advantage of using this type of data is that it can help to reduce bias in AI algorithms.
By incorporating human feedback into training datasets, we can ensure our algorithms are better able to replicate human behavior. In addition, qualitative data can also help us understand why certain algorithms produce certain results. This can be extremely valuable, as it allows our algorithms to become more accurate.
So, if you’re looking to create a dataset for a machine learning task, don’t forget about qualitative data! It could make all the difference.
Start growing with data and Twine AI
How Qualitative Datasets Benefit Real-life Machine Learning Projects
Case #1
Qualitative datasets are great for providing additional context for AI systems. One example of this would be Amazon’s Alexa Virtual Assistant.
When you ask the “Alexa” a question like “Who was president during World War Two?”, the AI device will answer your query whilst providing links to relevant Wikipedia entries. This is so you have access to more information if required (such as dates or locations).
However, Google Home doesn’t offer this same functionality. If you ask it the same question, it will simply answer with a list of presidents who served during World War Two without any contextual information. This is because Amazon has incorporated qualitative data into its assistant’s algorithms by adding additional text-based resources beyond just numerical data. This helps to improve accuracy and make the assistant more user-friendly.
Case #2
Qualitative data can also help to reduce training dataset size.
Take the example of image recognition: a typical machine learning image recognition algorithm requires millions of images in order to be accurately trained.
However, if we use qualitative data in addition to quantitative data, then we can reduce this number significantly. The reason for this is that qualitative data contains information about the context of an image, including things like who’s in a photo, what they’re wearing, and where it was taken. This additional contextual information allows us to replace many images with just one or two samples.
If we were training an algorithm using only quantitative data, for instance, we might require ten different photos of our friend Anna, in order to train it accurately to recognize her. By including this kind of data alongside these images (such as her name, hair color, smile, etc.) we could reduce those ten examples down to just one or two pictures instead.
Case #3
Qualitative data has been shown to be more effective than purely numerical datasets at replicating human behavior.
This is useful for situations where we can’t observe humans ourselves: like when a computer has to learn how to drive on its own. It also helps us understand why an algorithm produced certain results in the first place (and therefore how it could be improved).
For example, Amazon uses qualitative data from people who review products online and applies this information to train Alexa’s algorithms so that she understands what those reviews mean.
For queries like: “Alexa, do I need boots or shoes for hiking?”; you’ll get responses like: “I recommend waterproof hiking boots because they are better at protecting your feet from the cold water!”.
How to Obtain Qualitative Data for Your Own Machine Learning Projects
Online services, such as Google and Facebook, offer face recognition APIs that allow developers to train algorithms on millions of user-generated images. However, these APIs often require a significant amount of training data in order to produce accurate results. By incorporating qualitative data into the training dataset, we can reduce the number of images required significantly.
But how can you incorporate qualitative data into your next machine learning project? Twine can help.
From our global marketplace of over 450,000 diverse freelancers, we offer a wide range of customized qualitative datasets. These can be used for training AI vision and speech recognition algorithms, to understand human behavior. To get started, simply get in touch with us here and we’ll get to work, helping you find ethical datasets that suit your specific needs.
Start growing with data and Twine AI
Wrapping Up
By now, you probably understand why qualitative data is seen as the unsung hero of machine learning datasets. It supplements quantitative data and helps to improve the accuracy of models by providing additional context.
In vision and speech recognition tasks especially, this type of data can be used to create more realistic training datasets that better represent the real world. Not only will this improve the performance of artificial intelligence systems, but it will also make them more accurate, and able to handle more complex tasks.
So why not use qualitative data in your own machine learning projects?
The benefits are clear, and it’s easy to get started with twine.net/ai. To implement qualitative datasets and see improved accuracy in no time – reach out today.