
For more useful AI content, check out our blog…
One of the top priorities of businesses today is to transform their operations using artificial intelligence (AI) and machine learning (ML) capabilities. Many companies haven’t yet seen a significant impact from their AI/ML investments. So… what’s missing? A great data strategy.
A data strategy that’s ready for a world of artificial intelligence and machine learning.
If data for AI models is inaccessible, insufficient, biased, or just straight-up bad, you’re going to have a hard time succeeding with AI. But there’s a solution waiting for you: AI-first data strategy.
Before we delve into this strategy, let’s examine how inadequate data hinders AI adoption and success.
Want to get your AI-ready data? Explore ethically sourced datasets from Twine AI.
Three significant data barriers to AI adoption
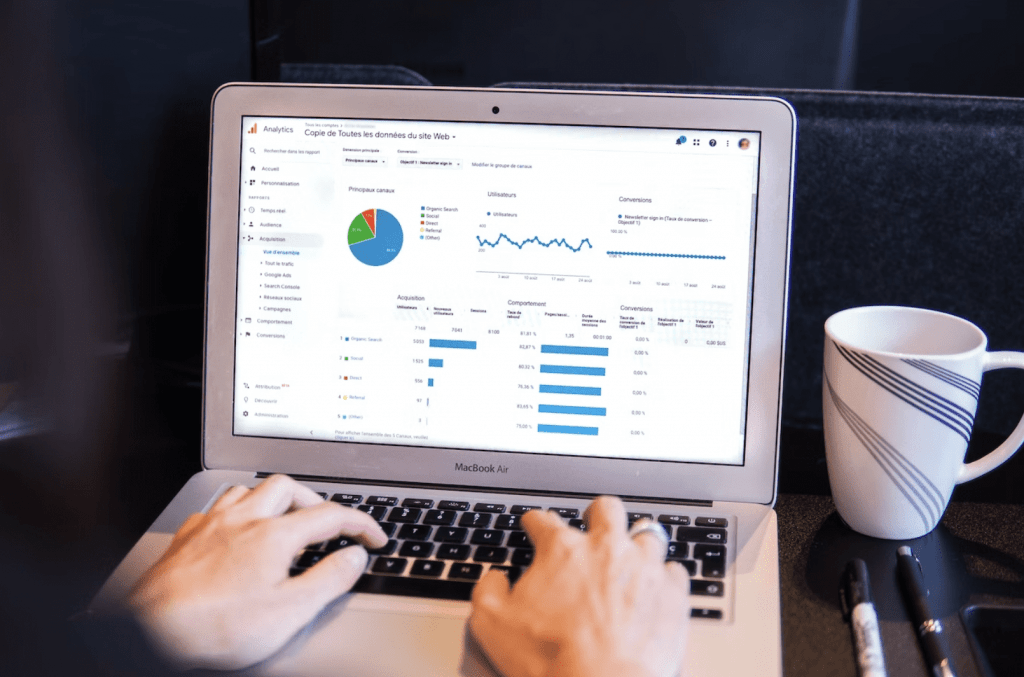
Remember the famous phrase “garbage in, garbage out” from the early computing days?
It holds true for AI and ML models, too. AI models are only as good as the data they receive from us, humans. Unfortunately, getting the data AI-ready is the biggest roadblock many businesses face when it comes to building a successful AI model.
Companies have bad data. Not all of it, but often it’s incomplete, mislabeled, or siloed in different departments. Operating an AI or ML model using this data produces poor results. Look at the common data issues plaguing AI projects.
Not enough data
For AI solutions to function correctly, you need large volumes of quality data to teach the underlying model.
For instance, if you want to train a multilingual natural language understanding (NLU) model, you need millions of bits of human speech in formats ML models can ingest. However, not all companies have the resources to capture or get such a great amount of datasets. 42% of companies find it very challenging to obtain the necessary data.
Sometimes a company has a lot of data, but it’s not helpful to the AI model they’re adopting. For instance, data scientist Henry Martinez explained that he once had to build an AI model to predict aircraft engine failure. While Martinez had information about turbine engines, other major aircraft components, and maintenance logs, he simply didn’t have any data on equipment failure.
He couldn’t create an AI model to predict equipment failure without data about equipment failure. The situation illustrates the problem of lack of data. Nearly a third of companies consider it the biggest bottleneck around their AI projects.
Lack of access
On average, businesses get data from about 20 different sources for their AI, business intelligence, and analytics. The volume of data an organization handles is growing at a staggering pace of 63% every month.
To manage all this data, most companies are using 4-6 data platforms or more, creating a sprawling data infrastructure. The data resides in various systems and formats without easy access and interoperability. In these cases, the data becomes a liability that leaves sensitive information unsecured and slows down AI development.
Over 70% of data scientists struggle to access all the data needed to run AI programs. 32% of executives complain about data silos in their companies. Four out of ten companies find data management the biggest bottleneck to their AI projects.
Poor quality data
Imagine you’re building a computer vision AI model to recognize colors in images, but your datasets to train the models show red as blue and blue as yellow. What would the outcome be? Not great.
77% of data professionals report facing quality issues with their data. The problem with that is AI and ML simply do not work if they’re trained and operated with faulty data. An AI model trained in incomplete, incorrect, or inappropriate data only produces unreliable results and negatively affects business decisions. Organizations lose 5% of their annual revenue due to underperforming AI programs that use low-quality data.
The solution to the AI data problem: AI-first data strategy
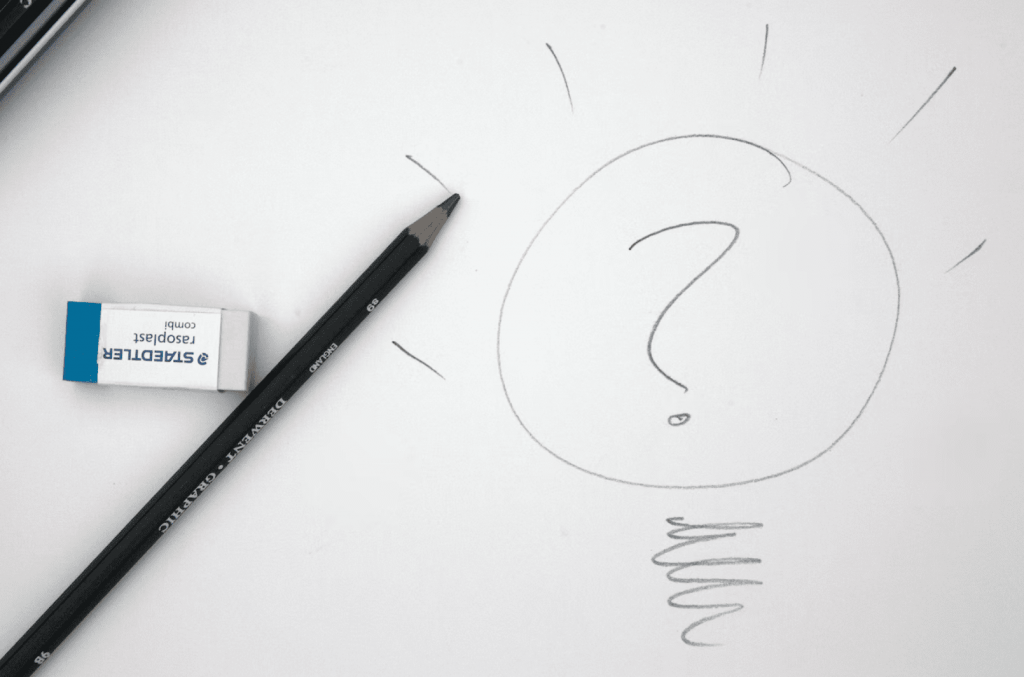
Adopting an AI-first data strategy sets you up for successful AI and ML projects. Every data strategy defines how and why data is captured. With the AI-first data strategy, a business intentionally collects, processes, and analyzes data for use in building AI and ML models.
What is an AI-first data strategy?
The AI-first data strategy lays the foundation for businesses to collect, organize, and analyze high-quality data for AI applications that support their business objectives.
It puts artificial intelligence at the center of a company’s data policy and outlines the long-term vision for the ways its data will reinforce AI and ML projects.
Shifting to the AI-first data strategy means you move your data strategy focus from centralized storage and data management to optimum data usage through AI models to increase sales, profit, and customer experience.
The importance of having an AI-first data strategy
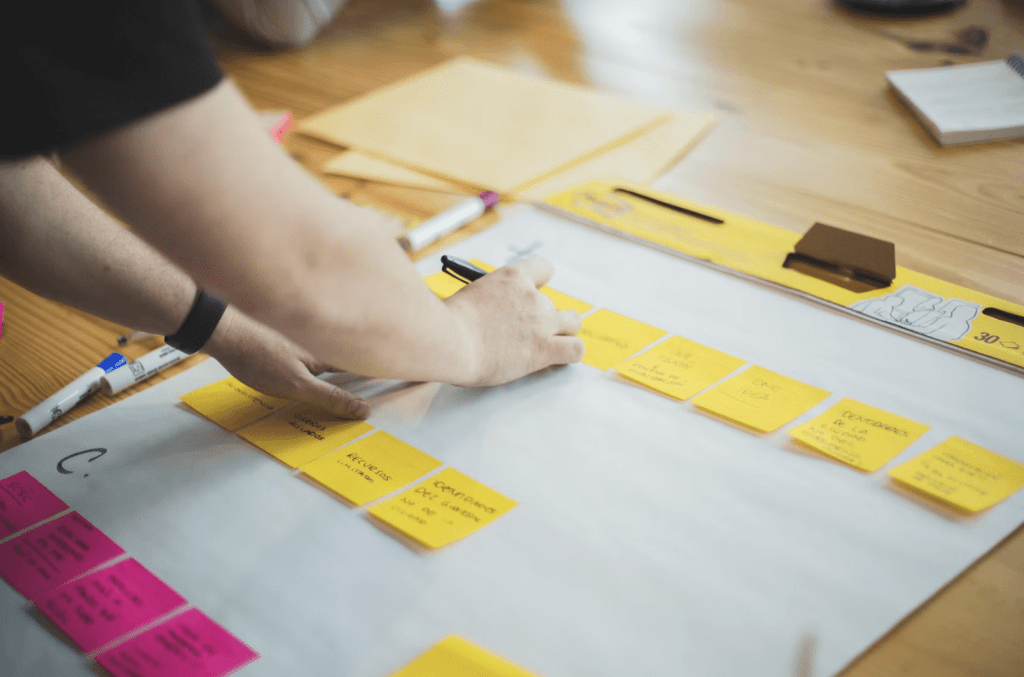
Today, companies are investing in AI at record levels. Still, only 2 out of 10 companies have established a data culture. 9 out of 10 organizations indicate that their data processes are still manual, resulting in highly skilled employees spending valuable time preparing and cleaning data.
Studies have shown that data scientists spend 60-80% of their time getting datasets ready when they could be training models or refining their algorithms. So not only are companies failing to harness the full potential of data with AI, they’re wasting their own resources and time.
Having an AI-first data strategy guides businesses with a data policy that completely unlocks the power of artificial intelligence. When implemented with the right tools and mindset, it takes care of each step of data prep.
How to approach the AI-first data strategy
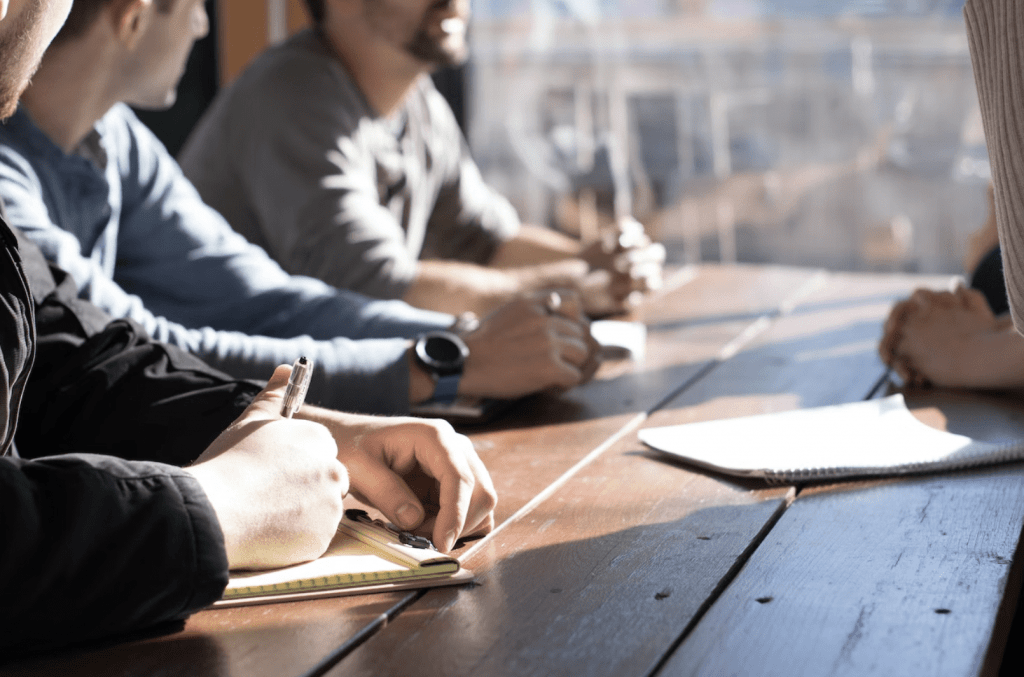
Your business can get your data AI-ready and successfully deploy AI projects with the AI-first data strategy. Find out how by following the below steps.
Discover your AI data needs
Companies need to get specific about what they want to achieve with their AI models. Diving into datasets before figuring out what you want from AI is like diving into the ocean to find a lost treasure. It might or might not exist.
Not exactly sure yet? Consider the following questions:
- What are your organization’s AI goals? Do you want to predict which customers will most likely renew their subscriptions? Do you want to use ML to offer the best product recommendations for your prospect?
- What is the most critical problem in your business process that an AI model could solve?
- What data is needed to build the required AI models?
If you don’t let your business goals from AI inform your data strategy, you could burn valuable time and resources collecting, storing, and analyzing the wrong data types.
Also, have you mastered data management? It’s the key to a 360° view of your enterprise data.
Find and source your AI data
Businesses typically acquire data internally and externally. Once you know what data you need for AI models and how you’ll use it, find out if you have the data you need internally.
If not, go the external route with third-party vendors. Multiple off-the-shelf AI data providers, like Twine AI, provide high-quality data at scale. Sourcing it from external vendors also makes it easy to ensure the data is high-quality, diverse, unbiased, and ethically sourced.
Not having ethically sourced data creates not only problems in AI models but also causes reputational, legal, and regulatory problems for the company. For instance, Clearview AI, a facial recognition company, has been fined in Italy and the United Kingdom for breaking laws regarding collecting online photos without consent.
Third-party vendors help avoid such problems as they collect data from required parties with informed consent and mask any private or sensitive data. They also collect data from diverse sources to avoid bias from the overrepresentation or underrepresentation of certain groups.
Some questions to start your AI data sourcing:
- What are your data sources for AI models?
- Will you need both external and internal datasets?
- What kind of data does your AI model need? Structured data, unstructured data, or a combination of both? What will be the key data attributes for your ML model?
- How will the data be collected?
- What data needs to be anonymized or encrypted?
- What is the expected volume and quality of required data?
Knowing the required volume, permissions, and quality guarantees your AI project doesn’t suffer because of insufficient data, ethical issues, or poor quality. Rely on the data operations (DataOps) team to streamline sourcing and create a data pipeline for AI initiatives. Use a data catalog tool to enforce data governance.
With an AI-first data strategy, the dataOps team also needs to be on the lookout for data that augments AI and ML models.
Organize and label your data
Labeled data is a must-have for AI models, especially for those with supervised learning. Organizations should build a process to curate, label, and certify data that’s ready for AI models.
Create guidelines for naming data and adding metadata to increase discoverability. If possible, companies ought to also lay out how to label data specifically for different AI use cases. Training for these tasks should be rigorous to reduce the number of errors made during this stage.
Once you have a process in place, you can use automated machine learning (AutoML) tools to automate the time-consuming and iterative annotation tasks.
Prepare your data
Data preparation makes it easy for AI models to ingest information. Data prep includes selecting desired features from datasets for AI models, along with normalizing and cleaning the datasets for missing inputs, inconsistencies, outliers, or other anomalies.
Pay special attention to the whole process of integrating, cleaning, enriching, and transforming datasets. Standardize the process wherever possible.
Most importantly, create guidelines for data scientists and ML engineers to share the data lineage transparently. They must share their data preparation and transformation processes so that others know the changes in data that flow into the AI model.
Ensure quality
High-quality datasets create high-quality AI models, so it’s imperative to ensure that your training datasets are complete, consistent, clean, and unbiased before it’s fed to AI models. The data science team should validate the prepared data and take corrective actions on any data gaps or forms of bias. Data engineers must address these issues from the onset as they have a high potential for negative effects.
For instance, Amazon pulled its AI recruitment engine a few years back because the data it trained on made it biased against women.
When you implement data governance policies to ensure data quality, they should include benchmarks to measure the accuracy and consistency of your datasets as well as the legal compliance of your data. If possible, enforce in-depth quality controls for each AI dataset when working on different AI models.
Train, test, and refine data models
Prepare your training data and test it. There’s no other way to ensure the data serves the ML models effectively. Divide your datasets into training data and a small subset of test data. Your test data has to be large enough to provide meaningful results, and it has to represent everything your AI needs to know.
After priming your model on the training datasets, validate it using the test dataset. Evaluate the results from the test datasets and tweak your model accordingly.
You can even divide your datasets into more than two parts to repeat the process and find the best model as you refine them. After all, iteration is essential for building AI models. In this stage, the best practice is to gather more data to refresh the test datasets rather than wearing out the models with available data.
Another critical step: track the code changes and results from the ML experiments. Keep note of parameters, features, and different versions of datasets used in the models for future reference. One way to do this is by bundling and storing the datasets, features used in ML models, and other model-related items using tools like feature stores and containers. These tools also provide easy, speedy access.
Deploy at scale
Once you have an AI model that’s passed your testing and validations, it’s time to deploy the project at scale. In this stage, the AI/ML model is typically packaged like a software unit to be deployed and used repeatedly. But it’s not easy.
Scaling AI requires collaboration between several different teams – DataOps, the ML operations (MLOps) team, IT, and other business stakeholders. The DataOps team needs to ensure that MLOps and the IT team have the required high-quality data to scale the AI model.
Observe, evaluate, and optimize
You’ve built a great model and launched it into production. But the work doesn’t stop here. AI applications have got to be frequently monitored, fine-tuned, and retrained as new real-time data streams in.
The scrutiny is vital because no business environment is static. Any change in the environment results in a change in data. When input changes, an AI model’s predictive power also changes. Data drift like this often leads to model decay.
Data science and the MLOps team should always watch out for any anomalies in the AI data pipeline that signal data drift. It is crucial to investigate what is causing the drift. Based on their analyses, you can retrain the model with new data or leave it as such and take it out of production until you get a solution. These proactive steps will prevent an AI failure due to any data drift.
Scale up
Getting your data AI-ready is not easy. So, lay a solid foundation with the AI-first data strategy. Get your data house in order. Collect, store, manage, and prepare your data with AI as a priority. Fearlessly design, test, deploy and review your data and AI projects. Treat data and AI as forever intertwined and set yourself up for success.
Want to get your AI-ready data? Explore ethically sourced datasets from Twine AI.
Ready to hire an expert? Our marketplace of over 500,000 diverse freelancers has the skills and expertise needed to skyrocket your business. From marketers to designers, copywriters to SEO experts – browse the talented bunch here!