AI has the potential to transform industries and solve complex problems, but it relies on datasets to learn and make predictions. In fact, the accuracy and reliability of the resulting machine learning model are directly related to the quality of the dataset and the power of AI.
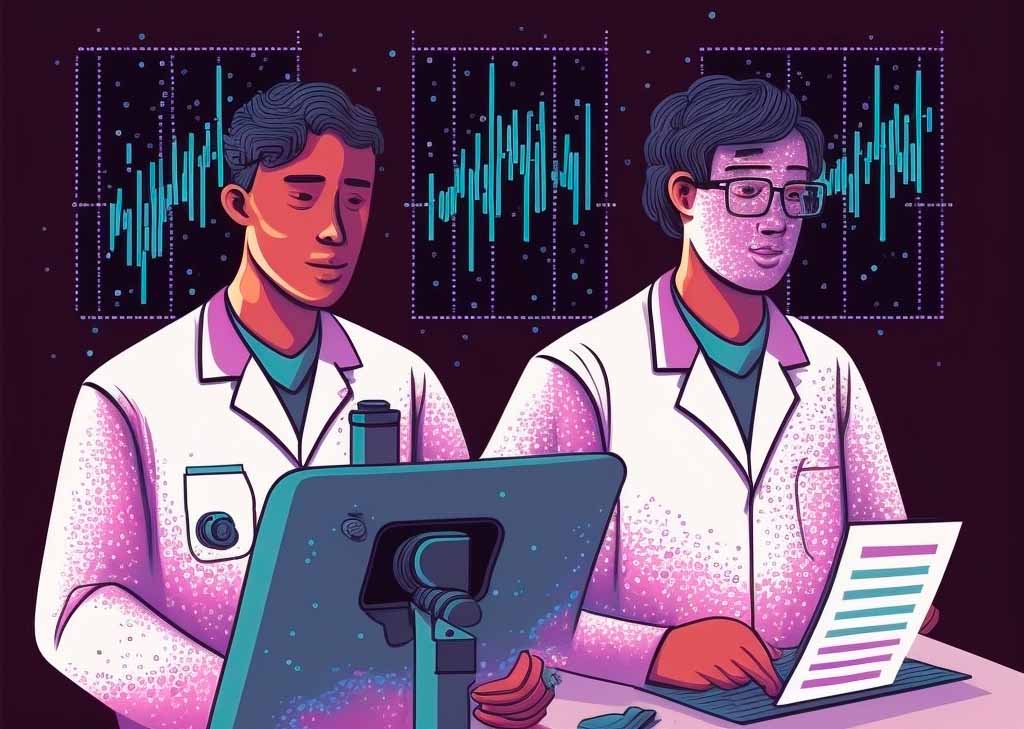
Using low-quality or poorly curated datasets can lead to many problems, such as bias, mistakes, and results that don’t matter. For example, let’s say that most of the pictures in a dataset used to train a facial recognition model are of white people. In that case, the model is likely to perform poorly when applied to images of other racial groups.
In the same way, if the dataset used to train a natural language processing model has typos and other mistakes, the model will have a hard time correctly processing and understanding text.
On the other hand, using high-quality datasets can result in more accurate and reliable results, better performance on real-world tasks, and greater confidence in the model’s predictions. Here are a few pointers to keep in mind to ensure that you are using the best datasets possible for your machine-learning projects:
Look for large and diverse datasets:
Machine learning algorithms work best when they are exposed to a wide range of data, so look for large datasets with a lot of different types of data. This will help the model learn more about the underlying patterns and relationships in the data and improve its ability to generalize to new situations.
Check the quality and relevance of the data:
Before using a dataset, make sure to evaluate its quality and relevance. Is the information complete and correct? Is it applicable to your particular task or domain? If the data is flawed or unrelated to your goals, your model’s performance will suffer.
Consider domain expertise:
If you’re working on a specific task or in a specific domain, using datasets curated or annotated by experts in that field can be beneficial. This can help you make sure that the data is useful and relevant to your goals.
Investigate preprocessing options:
Depending on what you want to do with your dataset, you might want to look into preprocessing options. This includes things like cleaning and normalizing the data, choosing the most important features, and using other types of data transformations to make the model work better.
Last but not least, the quality of the dataset has a big impact on how accurate and reliable machine learning models are. You can make it more likely that your AI projects will be successful by choosing high-quality datasets and taking domain expertise and preprocessing options into account. It appears that there is no upper bound to machine learning and the power of AI.